This AI could modify the short operating life of fusion reactors.
These experimental reactors are still a long way from being able to completely replace fossil fuels, despite the fact that scientists have been working towards the goal of achieving limitless and environmentally benign fusion electricity for decades. The unpredictability of superheated plasma has been a significant obstacle in the way of creating reliable power from fusion, but it appears that we may be one step closer to removing that obstacle from the list. An artificial intelligence (AI) capable of predicting the behaviour of plasma and smoothing out anomalies that could otherwise reset the reactor has been developed by a group of researchers from the Princeton Plasma Physics Laboratory (PPPL).
Nuclear fusion reactors are made up of a torus that resembles a donut and is used to heat hydrogen to extremely high temperatures. The plasma is contained within these “tokamak” reactors by magnetic fields, which prevent it from penetrating the walls of the torus it is contained within. However, the turbulent band of plasma does not remain in place for an extended period of time; at some point, what are known as “tearing mode instabilities” manifest themselves, which ultimately result in plasma escaping from the field. The reactor needs to be turned off and restarted at that point during the process. It is possible that the artificial intelligence that was built at PPPL will be able to forecast these instabilities just far enough in advance that they can be rectified.
The testing was carried out at the DIII-D National Fusion Facility in San Diego, which is owned and operated by the Department of Energy (see above). Since the 1980s, this tokamak reactor has been in operation; however, just like all other fusion reactors now in operation, it does not produce electricity that can be utilised. The fusion reaction can only be maintained for a few seconds by even the most successful reactors. In spite of this, the DIII-D system does make it possible for scientists to conduct experiments with nuclear fusion as we move closer to achieving viability. For the purpose of identifying indicators that plasma is going to erupt from the field, the researchers trained its artificial intelligence by using data from previous fusion tests conducted at this location.
“By learning from past experiments, rather than incorporating information from physics-based models, the AI could develop a final control policy that supported a stable, high-powered plasma regime in real time, at a real reactor,” stated principal investigator and PPPL physicist Egemen
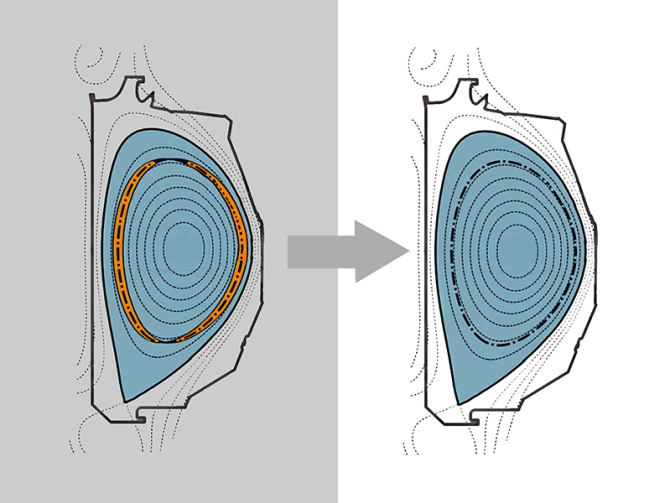
The warning that is generated by the AI system is not particularly lengthy; the maximum duration of the forecast is three hundred milliseconds. A human being would not be able to accomplish anything in that amount of time, but it just takes milliseconds for tearing mode instabilities to disrupt a fusion process. In order to stabilise the reaction, the neural network was able to learn which strategies were beneficial and then put those techniques into action at the appropriate time. The actions that are carried out by the artificial intelligence are frequently distinct from the set methods that a human operator might execute. The researchers have a hunch that if they could figure out why the artificial intelligence does certain things, it would let them learn more about the physics that are at play.
The group is certain that the artificial intelligence controller is operating properly in order to lessen the frequency of ripping mode instabilities in the DIII-D reactor. The network, on the other hand, was trained specifically on this system; hence, it will not be able to forecast or stabilise tearing mode instabilities in other tokamaks. In the long run, the researchers have the goal of developing a more universal artificial intelligence, which will require a great deal more testing. In addition to this, they anticipate expanding the capabilities of the AI controller so that it can simultaneously manage many control problems. “Nature” is the journal that has published the findings of the study.